Turn data science into business results
Posit makes it easy to deploy open source data science work across the enterprise safely and securely. Share Jupyter notebooks, Plotly dashboards, or interactive applications built with popular R and Python frameworks including Shiny, Streamlit, Dash, and Flask.
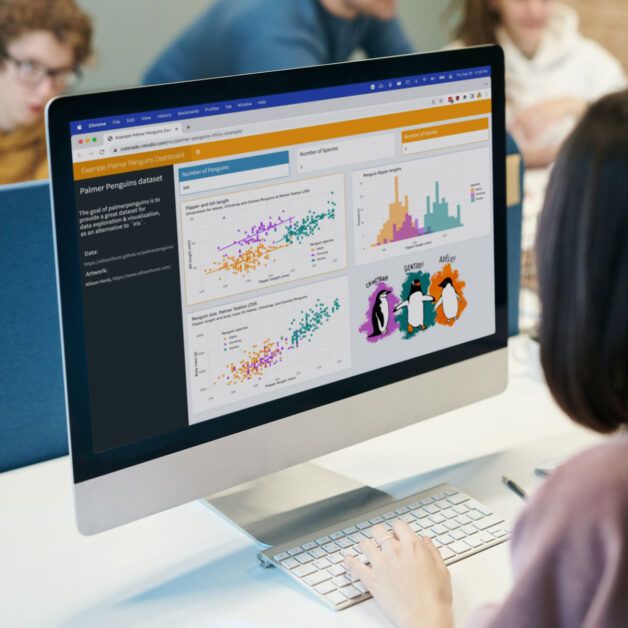
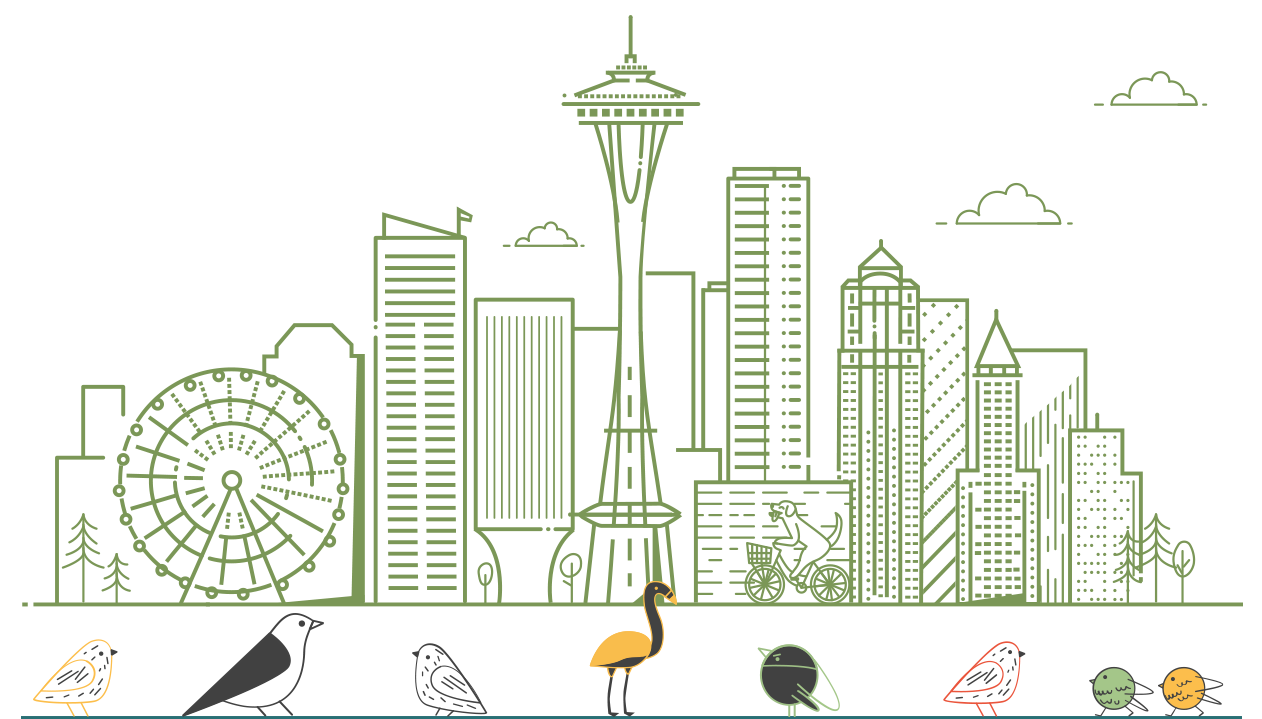
Scale open-source successfully
Thousands of professional data science teams rely on our solutions and expertise to get the most out of their investments in R and Python. We'd love to help you adopt open-source data science at scale with tools built for data scientists, by data scientists.
Enterprise for professionals
Securely share data-science applications
across your team—and the enterprise.
Open Source
Our code is your code. Build on it. Share it. Improve people’s lives with it.
Cloud Solutions
Take the time and effort out of uploading, storing, accessing, and sharing your work.
Our Solutions
We serve millions of users across all industries. Explore some of the ways our tools drive collaboration and build reproducible results.
Companies we work with
Trusted by 52 of the Fortune 100
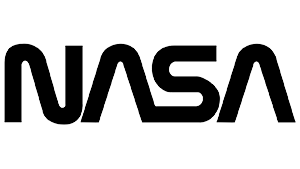
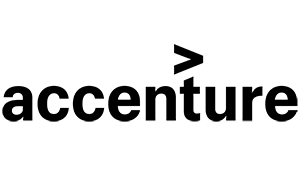
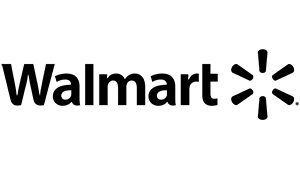
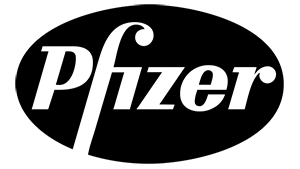
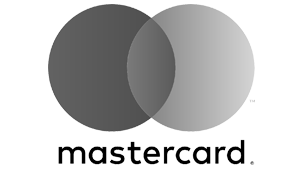
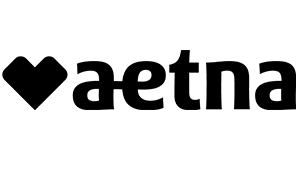
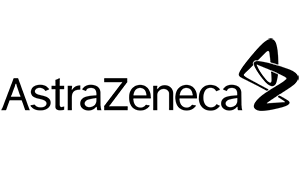
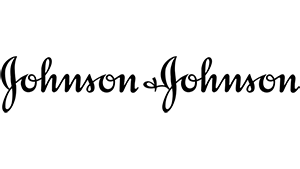
We’re always here to help
Welcome to a community that’s accessible, inclusive, and empowering. Just like our products.